Note
Go to the end to download the full example code
Drug Resistance Index (DRI
)
The Drug Resistance Index or DRI
measures changes through time in the proportion
of disease-causing pathogens that are resistant to the antibiotics commonly used to
treat them. The annual percentage change in the DRI
is a measure of the rate of
depletion of antibiotic effectiveness.
Since antibiotic use may change over time in response to changing levels of
antibiotic resistance, we compare trends in the index with the counterfactual
case, where antibiotic use remains fixed to a baseline year. A static-use DRI
allows assessment of the extent to which drug use has adapted in response to
resistance and the burden that this resistance would have caused if antibiotic
use patterns had not changed. Changing antibiotic use patterns over time may
mitigate the burden of antibiotic resistance. To incorporate changing trends
in antibiotic use, we also construct an adaptive version of the DRI
.
For more information see: pyamr.core.dri.DRI
21 # Libraries
22 import pandas as pd
23
24 # ----------------------------------
25 # Create data
26 # ----------------------------------
27 # Define susceptibility test records
28 susceptibility_records = [
29 ['2021-01-01', 'BLDCUL', 'ECOL', 'AAUG', 'sensitive'],
30 ['2021-01-01', 'BLDCUL', 'ECOL', 'AAUG', 'sensitive'],
31 ['2021-01-01', 'BLDCUL', 'ECOL', 'AAUG', 'sensitive'],
32 ['2021-01-01', 'BLDCUL', 'ECOL', 'AAUG', 'resistant'],
33 ['2021-01-02', 'BLDCUL', 'ECOL', 'AAUG', 'sensitive'],
34 ['2021-01-02', 'BLDCUL', 'ECOL', 'AAUG', 'sensitive'],
35 ['2021-01-02', 'BLDCUL', 'ECOL', 'AAUG', 'resistant'],
36 ['2021-01-03', 'BLDCUL', 'ECOL', 'AAUG', 'sensitive'],
37 ['2021-01-03', 'BLDCUL', 'ECOL', 'AAUG', 'resistant'],
38 ['2021-01-04', 'BLDCUL', 'ECOL', 'AAUG', 'resistant'],
39
40 ['2021-01-01', 'BLDCUL', 'ECOL', 'ACIP', 'sensitive'],
41 ['2021-01-01', 'BLDCUL', 'ECOL', 'ACIP', 'resistant'],
42 ['2021-01-01', 'BLDCUL', 'ECOL', 'ACIP', 'resistant'],
43 ['2021-01-01', 'BLDCUL', 'ECOL', 'ACIP', 'resistant'],
44 ['2021-01-02', 'BLDCUL', 'ECOL', 'ACIP', 'sensitive'],
45 ['2021-01-02', 'BLDCUL', 'ECOL', 'ACIP', 'resistant'],
46 ['2021-01-02', 'BLDCUL', 'ECOL', 'ACIP', 'resistant'],
47 ['2021-01-03', 'BLDCUL', 'ECOL', 'ACIP', 'sensitive'],
48 ['2021-01-03', 'BLDCUL', 'ECOL', 'ACIP', 'resistant'],
49 ['2021-01-04', 'BLDCUL', 'ECOL', 'ACIP', 'sensitive'],
50
51 ['2021-01-01', 'BLDCUL', 'SAUR', 'ACIP', 'resistant'],
52 ['2021-01-01', 'BLDCUL', 'SAUR', 'ACIP', 'resistant'],
53 ['2021-01-01', 'BLDCUL', 'SAUR', 'ACIP', 'resistant'],
54 ['2021-01-01', 'BLDCUL', 'SAUR', 'ACIP', 'resistant'],
55 ['2021-01-02', 'BLDCUL', 'SAUR', 'ACIP', 'sensitive'],
56 ['2021-01-02', 'BLDCUL', 'SAUR', 'ACIP', 'sensitive'],
57 ['2021-01-02', 'BLDCUL', 'SAUR', 'ACIP', 'resistant'],
58 ['2021-01-08', 'BLDCUL', 'SAUR', 'ACIP', 'sensitive'],
59 ['2021-01-08', 'BLDCUL', 'SAUR', 'ACIP', 'resistant'],
60 ['2021-01-08', 'BLDCUL', 'SAUR', 'ACIP', 'resistant'],
61 ['2021-01-08', 'BLDCUL', 'SAUR', 'ACIP', 'resistant'],
62 ['2021-01-08', 'BLDCUL', 'SAUR', 'ACIP', 'resistant'],
63 ['2021-01-08', 'BLDCUL', 'SAUR', 'ACIP', 'resistant'],
64 ['2021-01-09', 'BLDCUL', 'SAUR', 'ACIP', 'sensitive'],
65 ['2021-01-09', 'BLDCUL', 'SAUR', 'ACIP', 'sensitive'],
66 ['2021-01-09', 'BLDCUL', 'SAUR', 'ACIP', 'sensitive'],
67 ['2021-01-09', 'BLDCUL', 'SAUR', 'ACIP', 'sensitive'],
68 ['2021-01-09', 'BLDCUL', 'SAUR', 'ACIP', 'resistant'],
69
70 ['2021-01-12', 'URICUL', 'SAUR', 'ACIP', 'resistant'],
71 ['2021-01-12', 'URICUL', 'SAUR', 'ACIP', 'intermediate'],
72 ['2021-01-13', 'URICUL', 'SAUR', 'ACIP', 'resistant'],
73 ['2021-01-13', 'URICUL', 'SAUR', 'ACIP', 'sensitive'],
74 ['2021-01-14', 'URICUL', 'SAUR', 'ACIP', 'resistant'],
75 ['2021-01-14', 'URICUL', 'SAUR', 'ACIP', 'resistant'],
76 ['2021-01-15', 'URICUL', 'SAUR', 'ACIP', 'sensitive'],
77 ['2021-01-15', 'URICUL', 'SAUR', 'ACIP', 'sensitive'],
78 ['2021-01-16', 'URICUL', 'SAUR', 'ACIP', 'intermediate'],
79 ['2021-01-16', 'URICUL', 'SAUR', 'ACIP', 'intermediate'],
80 ]
81
82 # Define prescription test records
83 prescription_records = [
84 ['2021-01-01', 'PATIENT_1', 'AAUG', 150],
85 ['2021-01-02', 'PATIENT_1', 'AAUG', 221],
86 ['2021-01-03', 'PATIENT_1', 'AAUG', 152],
87
88 ['2021-01-01', 'PATIENT_2', 'AAUG', 254],
89 ['2021-01-02', 'PATIENT_2', 'AAUG', 325],
90 ['2021-01-03', 'PATIENT_2', 'AAUG', 356],
91
92 ['2021-01-01', 'PATIENT_3', 'ACIP', 457],
93 ['2021-01-02', 'PATIENT_3', 'ACIP', 428],
94 ['2021-01-03', 'PATIENT_3', 'ACIP', 459],
95
96 ['2021-01-01', 'PATIENT_4', 'ACIP', 50],
97 ['2021-01-02', 'PATIENT_4', 'ACIP', 50],
98 ['2021-01-03', 'PATIENT_4', 'ACIP', 50],
99
100 ]
101
102 # Create DataFrames
103 susceptibility = pd.DataFrame(susceptibility_records,
104 columns=['DATE',
105 'SPECIMEN',
106 'MICROORGANISM',
107 'ANTIMICROBIAL',
108 'SENSITIVITY'])
109
110 prescriptions = pd.DataFrame(prescription_records,
111 columns=['DATE',
112 'PATIENT',
113 'DRUG',
114 'DOSE'])
115
116 # Format dates
117 susceptibility.DATE = pd.to_datetime(susceptibility.DATE)
118 prescriptions.DATE = pd.to_datetime(prescriptions.DATE)
Lets see the susceptibility test records
122 susceptibility.head(5)
Lets see the prescription records
126 prescriptions.head(5)
Lets create the summary table and compute the DRI
132 # ------------------------
133 # Compute summary table
134 # ------------------------
135 # Libraries
136 from pyamr.core.sari import SARI
137
138 # Create sari instance
139 sari = SARI(groupby=['DATE',
140 'SPECIMEN',
141 'MICROORGANISM',
142 'ANTIMICROBIAL',
143 'SENSITIVITY'])
144
145 # Compute susceptibility summary table
146 smmry1 = sari.compute(susceptibility,
147 return_frequencies=False)
148
149 # Compute prescriptions summary table.
150 smmry2 = prescriptions \
151 .groupby(by=['DATE', 'DRUG']) \
152 .DOSE.sum().rename('use')
153
154 # Combine both summary tables
155 smmry = smmry1.reset_index().merge(
156 smmry2.reset_index(), how='inner',
157 left_on=['DATE', 'ANTIMICROBIAL'],
158 right_on=['DATE', 'DRUG']
159 )
160
161 # -------------------------
162 # Compute DRI
163 # -------------------------
164 # Librarie
165 from pyamr.core.dri import DRI
166
167 # Instance
168 obj = DRI(
169 column_resistance='sari',
170 column_usage='use'
171 )
172
173 # Compute DRI overall
174 dri1 = obj.compute(smmry)
175
176 # Compute DRI
177 dri2 = obj.compute(smmry,
178 groupby=['SPECIMEN'])
179
180 # Compute DRI
181 dri3 = obj.compute(smmry,
182 groupby=['MICROORGANISM'])
183
184 # Compute DRI
185 dri4 = obj.compute(smmry,
186 groupby=['MICROORGANISM', 'ANTIMICROBIAL'])
187
188 # Compute DRI
189 dri5 = obj.compute(smmry,
190 groupby=['DATE'],
191 return_usage=True)
192
193 # Compute DRI
194 dri6 = obj.compute(smmry,
195 groupby=['DATE', 'MICROORGANISM'],
196 return_usage=True)
197
198 # Compute DRI
199 dri7 = obj.compute(smmry,
200 groupby=['DATE', 'MICROORGANISM', 'ANTIMICROBIAL'],
201 return_usage=True,
202 return_complete=True)
203
204 # Compute DRI (return all elements of summary table).
205 dri8 = obj.compute(smmry,
206 groupby=['MICROORGANISM'],
207 return_complete=True)
208
209 # Show
210 print("\nDRI (1):")
211 print(dri1)
212 print("\nDRI (2):")
213 print(dri2)
214 print("\nDRI (3):")
215 print(dri3)
216 print("\nDRI (4):")
217 print(dri4)
218 print("\nDRI (5):")
219 print(dri5)
220 print("\nDRI (6):")
221 print(dri6)
222 print("\nDRI (7):")
223 print(dri7)
224 print("\nDRI (8):")
225 print(dri8)
DRI (1):
0.5478155956311913
DRI (2):
SPECIMEN
BLDCUL 0.5478
Name: dri, dtype: float64
DRI (3):
MICROORGANISM
ECOL 0.5049
SAUR 0.6765
Name: dri, dtype: float64
DRI (4):
MICROORGANISM ANTIMICROBIAL
ECOL AAUG 0.3683
ACIP 0.6382
SAUR ACIP 0.6765
Name: dri, dtype: float64
DRI (5):
use_period dri
DATE
2021-01-01 1418.0 0.6969
2021-01-02 1502.0 0.4394
2021-01-03 1017.0 0.5000
DRI (6):
use_period dri
DATE MICROORGANISM
2021-01-01 ECOL 911.0 0.5283
SAUR 507.0 1.0000
2021-01-02 ECOL 1024.0 0.4889
SAUR 478.0 0.3333
2021-01-03 ECOL 1017.0 0.5000
DRI (7):
DATE SPECIMEN MICROORGANISM ANTIMICROBIAL RESISTANCE DRUG USE use_period u_weight w_rate dri
DATE MICROORGANISM ANTIMICROBIAL
2021-01-01 ECOL AAUG 0 2021-01-01 BLDCUL ECOL AAUG 0.2500 AAUG 404 404 1.0 0.2500 0.2500
ACIP 1 2021-01-01 BLDCUL ECOL ACIP 0.7500 ACIP 507 507 1.0 0.7500 0.7500
SAUR ACIP 2 2021-01-01 BLDCUL SAUR ACIP 1.0000 ACIP 507 507 1.0 1.0000 1.0000
2021-01-02 ECOL AAUG 3 2021-01-02 BLDCUL ECOL AAUG 0.3333 AAUG 546 546 1.0 0.3333 0.3333
ACIP 4 2021-01-02 BLDCUL ECOL ACIP 0.6667 ACIP 478 478 1.0 0.6667 0.6667
SAUR ACIP 5 2021-01-02 BLDCUL SAUR ACIP 0.3333 ACIP 478 478 1.0 0.3333 0.3333
2021-01-03 ECOL AAUG 6 2021-01-03 BLDCUL ECOL AAUG 0.5000 AAUG 508 508 1.0 0.5000 0.5000
ACIP 7 2021-01-03 BLDCUL ECOL ACIP 0.5000 ACIP 509 509 1.0 0.5000 0.5000
DRI (8):
DATE SPECIMEN MICROORGANISM ANTIMICROBIAL RESISTANCE DRUG USE use_period u_weight w_rate dri
MICROORGANISM
ECOL 0 2021-01-01 BLDCUL ECOL AAUG 0.2500 AAUG 404 2952 0.1369 0.0342 0.5049
1 2021-01-01 BLDCUL ECOL ACIP 0.7500 ACIP 507 2952 0.1717 0.1288 0.5049
3 2021-01-02 BLDCUL ECOL AAUG 0.3333 AAUG 546 2952 0.1850 0.0617 0.5049
4 2021-01-02 BLDCUL ECOL ACIP 0.6667 ACIP 478 2952 0.1619 0.1079 0.5049
6 2021-01-03 BLDCUL ECOL AAUG 0.5000 AAUG 508 2952 0.1721 0.0860 0.5049
7 2021-01-03 BLDCUL ECOL ACIP 0.5000 ACIP 509 2952 0.1724 0.0862 0.5049
SAUR 2 2021-01-01 BLDCUL SAUR ACIP 1.0000 ACIP 507 985 0.5147 0.5147 0.6765
5 2021-01-02 BLDCUL SAUR ACIP 0.3333 ACIP 478 985 0.4853 0.1618 0.6765
Lets see the summary table
229 smmry
Lets see the sample 4
.
233 dri4.to_frame().round(decimals=3)
Lets see the sample 7
with return_components=True
.
237 dri7.rename(columns={
238 'MICROORGANISM': 'ORG',
239 'ANTIMICROBIAL': 'ABX'
240 }).round(decimals=3)
Lets see the sample 8
with return_complete=True
.
244 dri8.rename(columns={
245 'MICROORGANISM': 'ORG',
246 'ANTIMICROBIAL': 'ABX'
247 }).round(decimals=3)
Lets compute the fixed
index for comparison
252 # --------------------------------------------
253 # Compute DRI fixed
254 # --------------------------------------------
255 # Compute prescriptions on t0.
256 use_t0 = prescriptions \
257 .groupby(by=['DATE', 'DRUG']) \
258 .DOSE.sum().rename('use') \
259 .to_frame().reset_index() \
260 .groupby('DRUG').use.first()
261
262 # Add to summary table
263 smmry = smmry.assign(use_t0=smmry.DRUG.map(use_t0))
264
265 # Define groupby
266 groupby = [
267 'DATE',
268 'MICROORGANISM'
269 ]
270
271 # Compute DRI
272 dri9a = obj.compute(smmry,
273 groupby=groupby,
274 return_usage=True)
275
276 # Compute DRI using new USE
277 dri9b = obj.compute(smmry,
278 groupby=groupby,
279 return_usage=True,
280 column_usage='use_t0')
281
282 # aux = pd.concat([dri9a, dri9b], axis=1)
283 aux = dri9a.merge(dri9b,
284 left_index=True, right_index=True,
285 suffixes=['', '_fixed'])
286
287 # Concatenate (series)
288 # aux = pd.concat([
289 # dri9a.rename('dri'),
290 # dri9b.rename('dri_fixed')], axis=1)
291
292 # Show
293 print("\n\n")
294 print("\nSummary (variable):")
295 print(smmry)
296 print("\nDRI (9):")
297 print(aux)
Summary (variable):
DATE SPECIMEN MICROORGANISM ANTIMICROBIAL sari DRUG use use_t0
0 2021-01-01 BLDCUL ECOL AAUG 0.2500 AAUG 404 404
1 2021-01-01 BLDCUL ECOL ACIP 0.7500 ACIP 507 507
2 2021-01-01 BLDCUL SAUR ACIP 1.0000 ACIP 507 507
3 2021-01-02 BLDCUL ECOL AAUG 0.3333 AAUG 546 404
4 2021-01-02 BLDCUL ECOL ACIP 0.6667 ACIP 478 507
5 2021-01-02 BLDCUL SAUR ACIP 0.3333 ACIP 478 507
6 2021-01-03 BLDCUL ECOL AAUG 0.5000 AAUG 508 404
7 2021-01-03 BLDCUL ECOL ACIP 0.5000 ACIP 509 507
DRI (9):
use_period dri use_period_fixed dri_fixed
DATE MICROORGANISM
2021-01-01 ECOL 911.0 0.5283 911.0 0.5283
SAUR 507.0 1.0000 507.0 1.0000
2021-01-02 ECOL 1024.0 0.4889 911.0 0.5188
SAUR 478.0 0.3333 507.0 0.3333
2021-01-03 ECOL 1017.0 0.5000 911.0 0.5000
Lets see the new summary (added old use column <old> for comparison)
302 smmry
Lets see the results for both fixed and dynamic.
306 aux
Lets visualise it
311 # --------------------------
312 # Plot
313 # --------------------------
314 # Libraries
315 import matplotlib.pyplot as plt
316 import seaborn as sns
317
318 # Filter
319 stacked = aux[['dri', 'dri_fixed']].stack().reset_index()
320
321 # Display using relplot
322 sns.relplot(data=stacked,
323 x='DATE', y=0, row='MICROORGANISM', hue='level_2',
324 # hue='event', style='event', col='region', palette='palette',
325 #height=4, aspect=2.0,
326 kind='line', linewidth=1.00, markersize=6, marker='o'
327 )
328
329 # Show
330 plt.show()
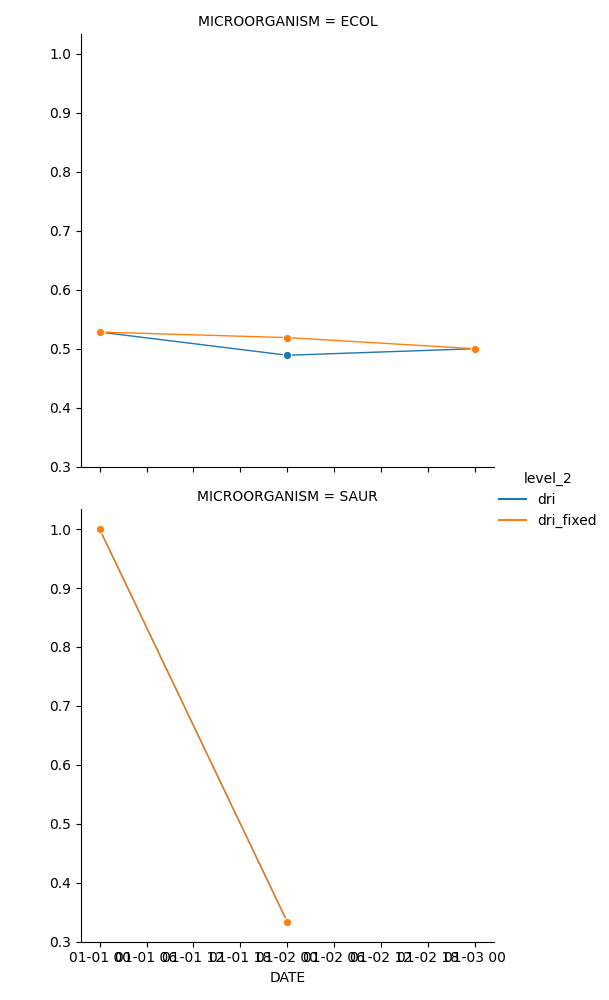
Note
The summary table for the prescriptions data computed above
aggregated the values in the column dose
which indicates the
specific dose delivered to the patient. In many scenarios, this
information might not be available or dosage units might vary (e.g.
mg or ml). A possible workaround is to count the number of
entries in the prescriptions data by using DOSE.count()
but
this workaround should be use with caution.
Total running time of the script: ( 0 minutes 0.649 seconds)